
Knowing this early on certainly saves you time, money, and frustration! Looking at bias metrics computed by SageMaker Clarify on your dataset, you can then add your own bias reduction techniques to your data processing pipeline. Indeed, a heavily biased dataset may well be unsuitable for training. Let’s look at each of these capabilities.ĭetecting dataset bias: This is an important first step. Detect bias drift and feature importance drift over time, thanks to the integration with Amazon SageMaker Model Monitor.Explain how feature values contribute to the predicted outcome, both for the model overall and for individual predictions.Measure bias using a variety of statistical metrics.Detect bias in datasets prior to training, and in models after training.Thanks to SageMaker Clarify, data scientists are able to: It’s integrated with SageMaker Studio, our web-based integrated development environment for ML, as well as with other SageMaker capabilities like Amazon SageMaker Data Wrangler, Amazon SageMaker Experiments, and Amazon SageMaker Model Monitor. SageMaker Clarify is a new set of capabilities for Amazon SageMaker, our fully managed ML service. We got to work, and came up with SageMaker Clarify. Thus, our customers asked us for help on detecting bias in their datasets and their models, and on understanding how their models make predictions. In addition, some regulations may require explainability when ML models are used as part of consequential decision making, and closing the loop, explainability can also help detect bias. Many companies and organizations may need ML models to be explainable before they can be used in production. Just like the prehistoric tribes in Stanley Kubrick’s “2001: A Space Odyssey,” we’re often left staring at an impenetrable monolith and wondering what it all means.

However, as models become more and more complex (I’m staring at you, deep learning), this kind of analysis becomes impossible. You can then decide whether this process is consistent with your business practices, basically saying: “yes, this is how a human expert would have done it.”
CLARIFY IMAGE CRACK
For simple and well-understood algorithms like linear regression or tree-based algorithms, it’s reasonably easy to crack the model open, inspect the parameters that it learned during training, and figure out which features it predominantly uses. Now, let’s discuss the explainability problem. It is thus important for model administrators to be aware of potential sources of bias in production systems. Unfortunately, even with the best of intentions, bias issues may exist in datasets and be introduced into models with business, ethical, and regulatory consequences. Under-representation for such groups could result in a disproportionate impact on their predicted outcomes. In fact, some of these groups may correspond to various socially sensitive features such as gender, age range, or nationality. As the number of classes, features, and unique feature values increase, your dataset may only contain a tiny number of training instances for certain groups. There are many variants of this under-representation problem. In fact, a trivial model could simply decide that transactions are always legitimate: as useless as this model would be, it would still be right 99.9% of the time! This simple example shows how careful we have to be about the statistical properties of our data, and about the metrics that we use to measure model accuracy. fraudulent), there’s a strong chance that it would be strongly influenced or biased by the majority group. Training a binary classification model (legitimate vs. Fortunately, the huge majority of transactions are legitimate, and they make up 99.9% of your dataset, meaning that you only have 0.1% fraudulent transactions, say 100 out of 100,000. Imagine that you’re working on a model detecting fraudulent credit card transactions.
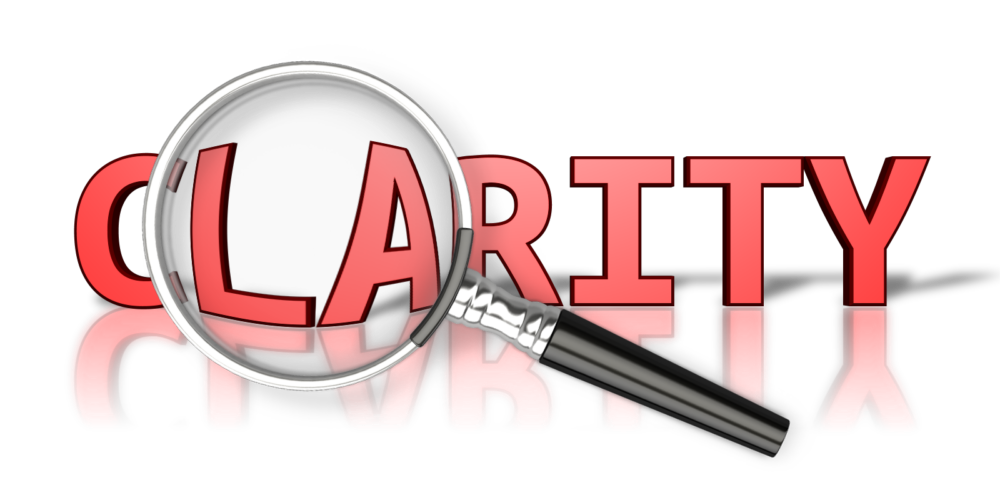
They are very real, and their implications can be far-reaching. First, can we ever hope to explain why our ML model comes up with a particular prediction? Second, what if our dataset doesn’t faithfully describe the real-life problem we were trying to model? Could we even detect such issues? Would they introduce some sort of bias in imperceptible ways? As we will see, these are not speculative questions at all. Today, I’m extremely happy to announce Amazon SageMaker Clarify, a new capability of Amazon SageMaker that helps customers detect bias in machine learning (ML) models, and increase transparency by helping explain model behavior to stakeholders and customers.Īs ML models are built by training algorithms that learn statistical patterns present in datasets, several questions immediately come to mind.
